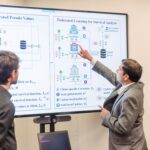
Today, a plethora of technologies, from image recognition tools to chatbots, are powered by machine learning. A key component to the technique’s success is data—and lots of it. For doctors and hospitals who hope to use machine learning to improve healthcare, that need for copious data presents a problem: medical data is protected by privacy laws and often exists in incomplete or diverse forms—from doctor’s notes to medical scans—that make it difficult for machine learning models to use it effectively.
“Using data better to aid medical decisions is a grand challenge of the 21st century,” says Sanjay Purushotham, an assistant professor in information systems at UMBC. “We need innovations in existing techniques to take full advantage of artificial intelligence in healthcare.”
Together with his students, Purushotham is tackling that challenge. He recently received a prestigious NSF CAREER award to support his team’s efforts to develop new ways to train health-focused machine learning models.
Purushotham has been contributing his expertise in computer science to collaborations with doctors and hospitals for almost ten years. The NSF CAREER award will help Purushotham further that research in a new direction, and ultimately, his team hopes their work will improve medical treatments and reduce costs, benefiting patients around the world.
The need for data-driven healthcare
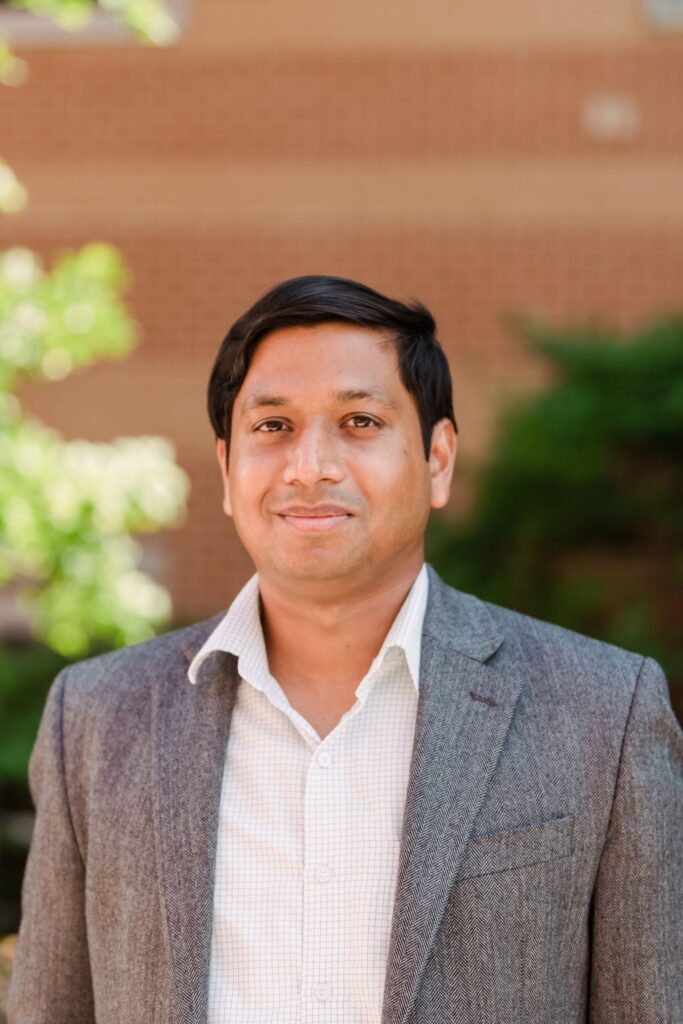
When you visit the doctor, you may be asked about your general health, your family medical history, and your current symptoms. The doctor may order a series of tests and scans. All of this data could yield valuable insights into your health and the best course of medical treatment.
Bringing the data together in ways that make it accessible and illuminating is an ongoing challenge in health care. Synthesizing and presenting health information in new ways could improve individual medical care. Computer systems could ensure busy doctors see the most relevant information at the right time, aiding their decision making. It could also prove valuable for addressing public health challenges, such as the spread of infectious diseases.
“During the COVID pandemic, the health community realized the importance of having access to good data,” says Purushotham. Better data could help public officials make better decisions about when to take precautions, such as closing schools. It could also help individuals better understand the risks when choosing to partake in activities like attending large social gatherings or traveling.
Over the next five years, the award will support Purushotham and his students as they investigate ways to advance a technique called federated learning, which could allow hospitals and doctors to jointly build and evaluate a machine learning model without sharing sensitive medical data.
A new way to collaborate
Most machine learning models are trained centrally, on vast quantities of data that are often scrapped from the internet. However, a lot of data exists that cannot be sent to a central processing facility, for cost or privacy reasons. The term ‘federated learning’ was coined in 2016 to describe a decentralized machine learning technique that could train on data that never leaves users’ mobile phones. Instead, individual devices would take turns downloading the model, training it on their own data, and then sending the updated model parameters back to a central location.
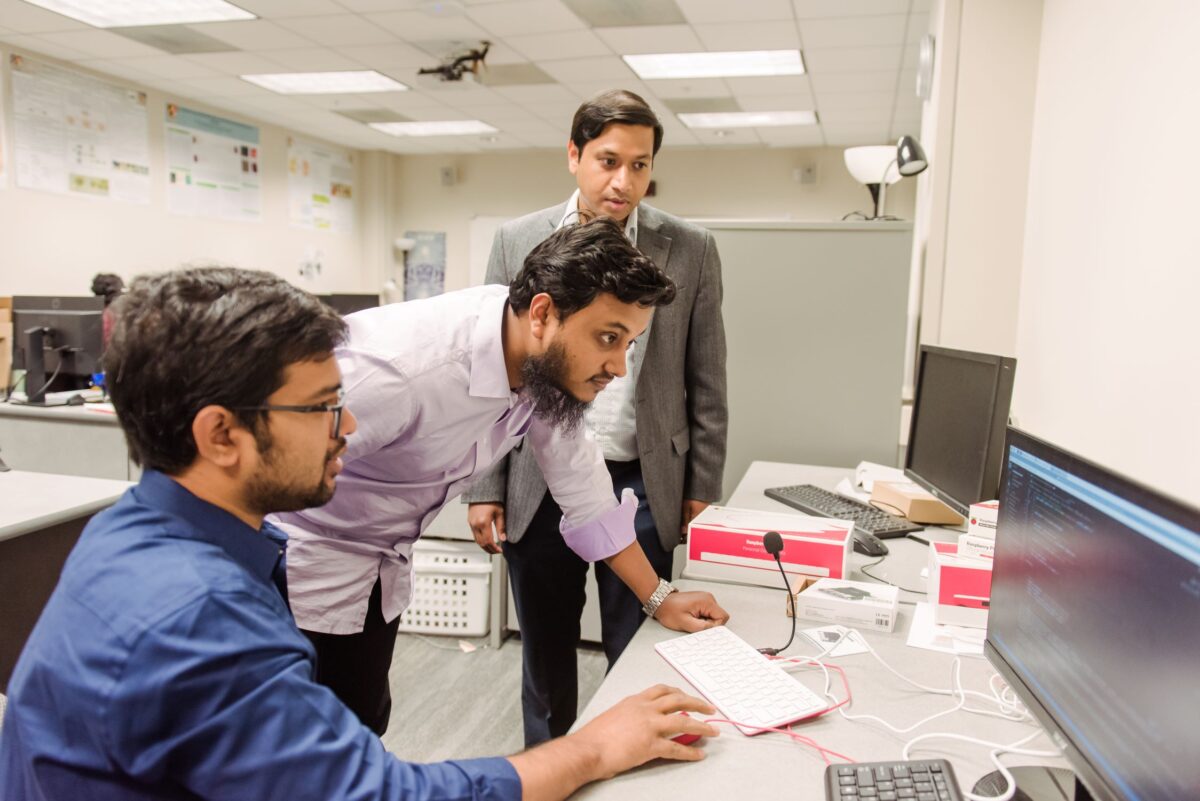
The general approach could be beneficial in many industries, from finance to manufacturing. In health care, federated learning has clear appeal because privacy laws strictly limit how health data can be shared.
For example, federated learning could allow hospitals to train a joint model on their medical data, such as CT scans, without sharing the scans with each other. The jointly trained model might reveal new ways to detect disease.
Yet, many hurdles remain to successfully deploying the technique, including the non-uniformity and limited nature of some patient data, the varying computational resources available to different medical practitioners, and the threat of bad actors seeking access to private information.
Advancing federated learning
In the coming years, Purushotham and his team will pursue three main avenues of research to address the obstacles of deploying federated learning in healthcare. The project will develop new algorithms, methodologies, and software to make data-driven federated learning for healthcare more robust and trustworthy.
The first focus of the team will be to develop new ways to handle the diversity of health data. The team will then study potential methods of attacks on the federated learning systems, and develop defenses against such attacks. The researchers will also investigate ways that the system can “un-learn” in situations where users request the influence of their data be removed from the model. In all cases, the researchers will focus on developing fair and interpretable algorithms. Finally, the researchers will study ways to generate synthetic health data, which can be used to augment or replace real data to improve the AI models.
The scope of the work is ambitious, but Purushotham is confident in his team.
“I have great students and collaborators,” he says. “I’m really excited to make federated learning in healthcare work.”
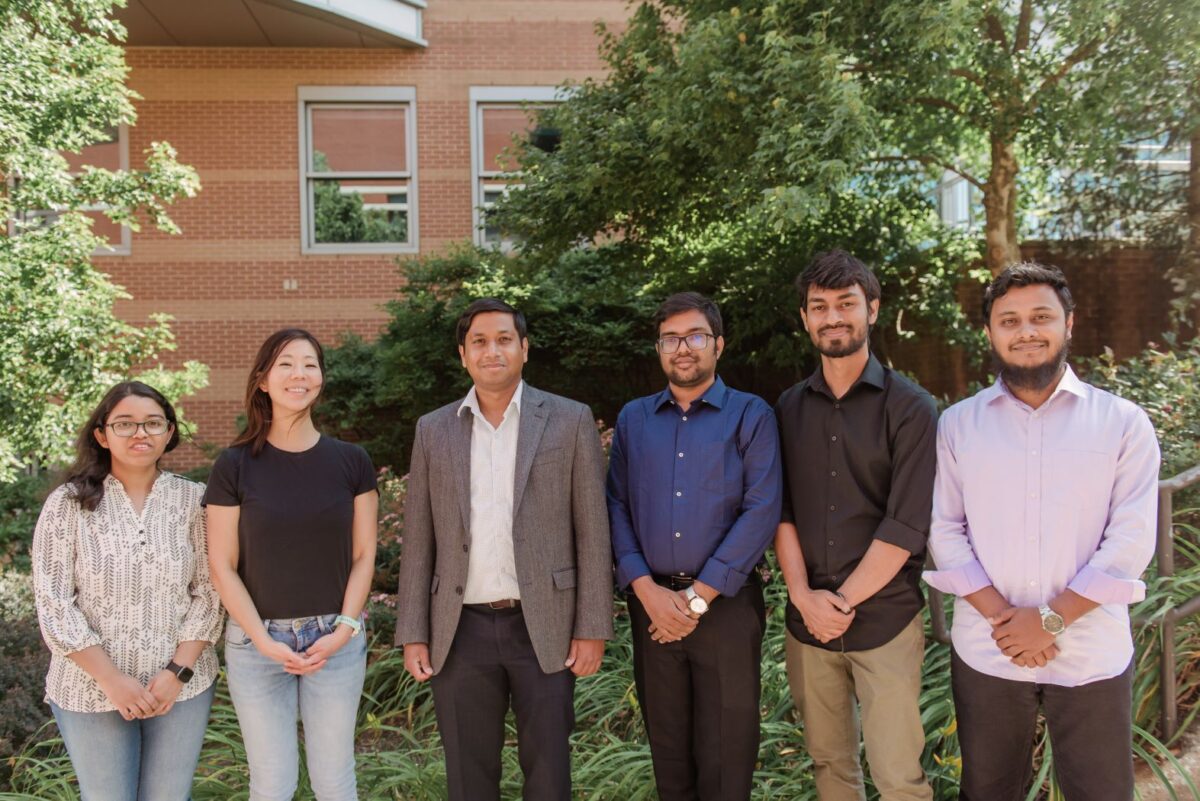